What Twitter learned from the Recsys 2020 Challenge [Michael Bronstein et al.]
‘What Twitter learned from the Recsys 2020 Challenge’ is a blog post authored by Michael Bronstein, Luca Belli, Apoorv Sharma, Yuanpu Xie, Ying Xiao, Dan Shiebler, Max Hansmire, and Wenzhe Shi; published on TowardsDataScience.
The authors describe the RecSys 2020 Challenge that was performed on Twitter data.
[We] describe the dataset and the three winning entries submitted by Nvidia, Learner, and Wantely teams. We try to make general conclusions about the choices that helped the winners achieve their results, notably: most important features; extremely fast experimentation speed for feature selection and model training; adversarial validation for generalisation; use of content features; use of decision trees over neural networksWe hope that these findings will be useful to the wider research community and inspire future research directions in recommender systems.
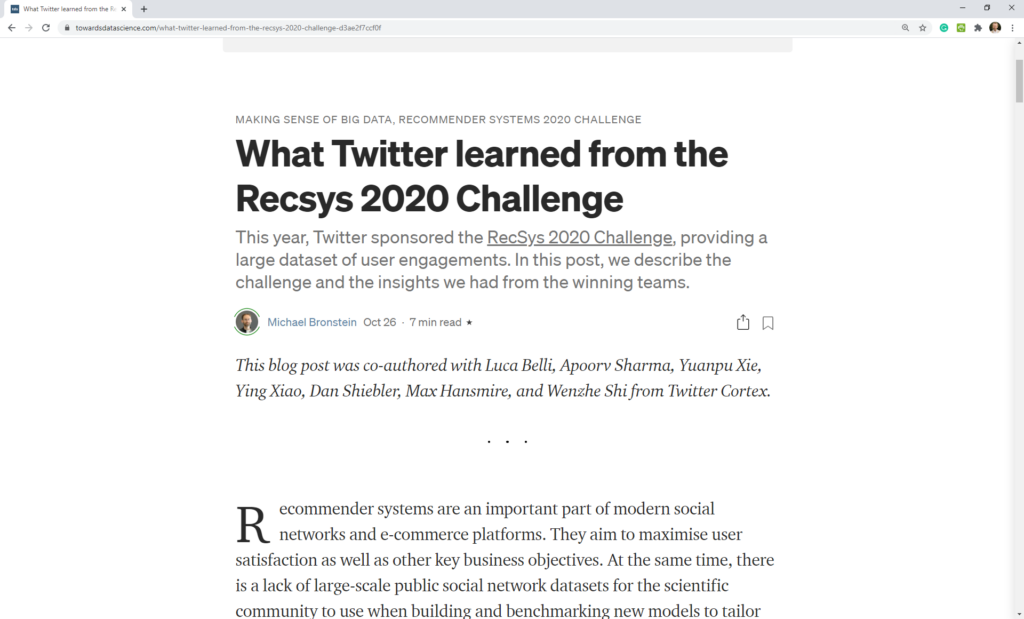
Related Posts
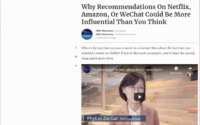
Why Recommendations On Netflix, Amazon, Or WeChat Could Be More Influential Than You Think [Forbes]
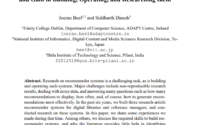
Real-World Recommender Systems for Academia: The Pain and Gain in Building, Operating, and Researching them [Beel & Dinesh]
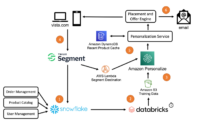
Case Study on how VistaPrint uses Amazon Personalize for product recommendations
About The Author
Joeran Beel
I am the founder of Recommender-Systems.com and head of the Intelligent Systems Group (ISG) at the University of Siegen, Germany https://isg.beel.org. We conduct research in recommender-systems (RecSys), personalization and information retrieval (IR) as well as on automated machine learning (AutoML), meta-learning and algorithm selection. Domains we are particularly interested in include smart places, eHealth, manufacturing (industry 4.0), mobility, visual computing, and digital libraries. We founded or maintain, among others, LensKit-Auto, Darwin & Goliath, Mr. DLib, and Docear, each with thousand of users; we contributed to TensorFlow, JabRef and others; and we developed the first prototypes of automated recommender systems (AutoSurprise and Auto-CaseRec) and Federated Meta Learning (FMLearn Server and Client).