CfP ACM TOIS: Special Section on Causality Representation Learning in LLMs-Driven Recommender Systems
The ACM Transactions on Information Systems (TOIS) announces a special section dedicated to “Causality Representation Learning in LLMs-Driven Recommender Systems.” This call for papers invites researchers to explore the intersection of causal inference and recommender systems, particularly focusing on the role of Large Language Models (LLMs) in enhancing causal discovery and system transparency.
The use of deep learning in recommender systems has grown rapidly. These systems excel in predicting user preferences but often lack interpretability. The application of causal inference can address this gap, making systems more transparent and reliable. LLMs provide an exciting new opportunity to delve deeper into causal relationships, opening new research avenues.
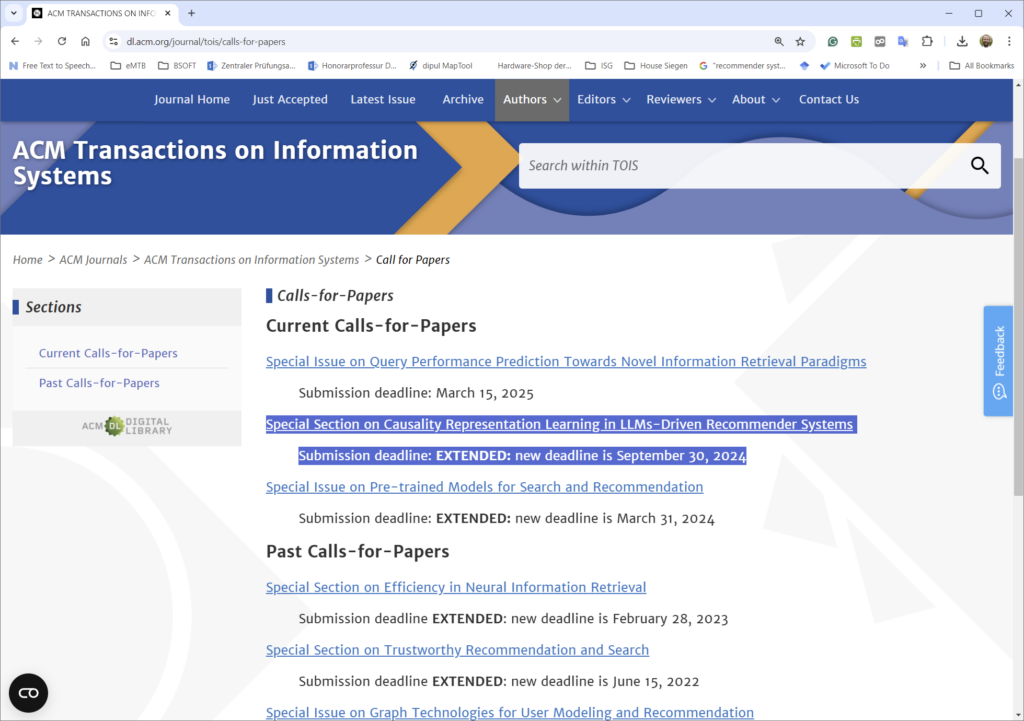
Topics of Interest: (excerpt)
- Causal inference foundations in recommender systems.
- Methods for estimating causal effects in user-item interactions.
- Causal relationships in interpretability and transparency.
- Interventions and their effects in recommender systems.
- Bias, fairness, and causal robustness.
- Applications of LLMs in causal discovery.
The guest editors are established experts from institutions such as CSIRO’s Data61, University of New South Wales, University of California San Diego, Rutgers University, and Carnegie Mellon University.
Submission Deadlines:
- Deadline: September 30, 2024
- First Notification: November 30, 2024
- Final Decision: April 30, 2025
This special section provides a platform for researchers to contribute to the understanding and development of causality in recommender systems, addressing both theoretical and practical challenges.
Full details: https://dl.acm.org/journal/tois/calls-for-papers